By Sibel Eker, IIASA postdoctoral research scholar
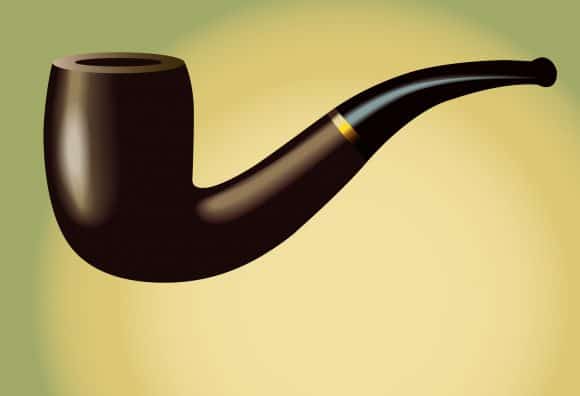
Ceci n’est pas une pipe – This is not a pipe © Jaka Vukotič | Dreamstime.com
Quantitative models are an important part of environmental and economic research and policymaking. For instance, IIASA models such as GLOBIOM and GAINS have long assisted the European Commission in impact assessment and policy analysis2; and the energy policies in the US have long been guided by a national energy systems model (NEMS)3.
Despite such successful modelling applications, model criticisms often make the headlines. Either in scientific literature or in popular media, some critiques highlight that models are used as if they are precise predictors and that they don’t deal with uncertainties adequately4,5,6, whereas others accuse models of not accurately replicating reality7. Still more criticize models for extrapolating historical data as if it is a good estimate of the future8, and for their limited scopes that omit relevant and important processes9,10.
Validation is the modeling step employed to deal with such criticism and to ensure that a model is credible. However, validation means different things in different modelling fields, to different practitioners and to different decision makers. Some consider validity as an accurate representation of reality, based either on the processes included in the model scope or on the match between the model output and empirical data. According to others, an accurate representation is impossible; therefore, a model’s validity depends on how useful it is to understand the complexity and to test different assumptions.
Given this variety of views, we conducted a text-mining analysis on a large body of academic literature to understand the prevalent views and approaches in the model validation practice. We then complemented this analysis with an online survey among modeling practitioners. The purpose of the survey was to investigate the practitioners’ perspectives, and how it depends on background factors.
According to our results, published recently in Eker et al. (2018)1, data and prediction are the most prevalent themes in the model validation literature in all main areas of sustainability science such as energy, hydrology and ecosystems. As Figure 1 below shows, the largest fraction of practitioners (41%) think that a match between the past data and model output is a strong indicator of a model’s predictive power (Question 3). Around one third of the respondents disagree that a model is valid if it replicates the past since multiple models can achieve this, while another one third agree (Question 4). A large majority (69%) disagrees with Question 5, that models cannot provide accurate projects, implying that they support using models for prediction purposes. Overall, there is no strong consensus among the practitioners about the role of historical data in model validation. Still, objections to relying on data-oriented validation have not been widely reflected in practice.
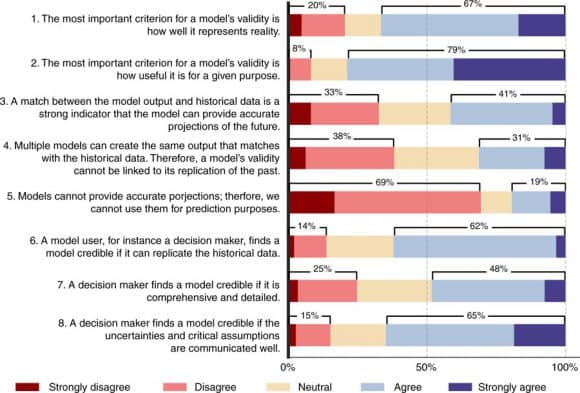
Figure 1: Survey responses to the key issues in model validation. Source: Eker et al. (2018)
According to most practitioners who participated in the survey, decision-makers find a model credible if it replicates the historical data (Question 6), and if the assumptions and uncertainties are communicated clearly (Question 8). Therefore, practitioners think that decision makers demand that models match historical data. They also acknowledge the calls for a clear communication of uncertainties and assumptions, which is increasingly considered as best-practice in modeling.
One intriguing finding is that the acknowledgement of uncertainties and assumptions depends on experience level. The practitioners with a very low experience level (0-2 years) or with very long experience (more than 10 years) tend to agree more with the importance of clarifying uncertainties and assumptions. Could it be because a longer engagement in modeling and a longer interaction with decision makers help to acknowledge the necessity of communicating uncertainties and assumptions? Would inexperienced modelers favor uncertainty communication due to their fresh training on the best-practice and their understanding of the methods to deal with uncertainty? Would the employment conditions of modelers play a role in this finding?
As a modeler by myself, I am surprised by the variety of views on validation and their differences from my prior view. With such findings and questions raised, I think this paper can provide model developers and users with reflections on and insights into their practice. It can also facilitate communication in the interface between modelling and decision-making, so that the two parties can elaborate on what makes their models valid and how it can contribute to decision-making.
Model validation is a heated topic that would inevitably stay discordant. Still, one consensus to reach is that a model is a representation of reality, not the reality itself, just like the disclaimer of René Magritte that his perfectly curved and brightly polished pipe is not a pipe.
References
- Eker S, Rovenskaya E, Obersteiner M, Langan S. Practice and perspectives in the validation of resource management models. Nature Communications 2018, 9(1): 5359. DOI: 10.1038/s41467-018-07811-9 [pure.iiasa.ac.at/id/eprint/15646/]
- EC. Modelling tools for EU analysis. 2019 [cited 16-01-2019]Available from: https://ec.europa.eu/clima/policies/strategies/analysis/models_en
- EIA. ANNUAL ENERGY OUTLOOK 2018: US Energy Information Administration; 2018. https://www.eia.gov/outlooks/aeo/info_nems_archive.php
- The Economist. In Plato’s cave. The Economist 2009 [cited]Available from: http://www.economist.com/node/12957753#print
- The Economist. Number-crunchers crunched: The uses and abuses of mathematical models. The Economist. 2010. http://www.economist.com/node/15474075
- Stirling A. Keep it complex. Nature 2010, 468(7327): 1029-1031. https://doi.org/10.1038/4681029a
- Nuccitelli D. Climate scientists just debunked deniers’ favorite argument. The Guardian. 2017. https://www.theguardian.com/environment/climate-consensus-97-per-cent/2017/jun/28/climate-scientists-just-debunked-deniers-favorite-argument
- Anscombe N. Models guiding climate policy are ‘dangerously optimistic’. The Guardian 2011 [cited]Available from: https://www.theguardian.com/environment/2011/feb/24/models-climate-policy-optimistic
- Jogalekar A. Climate change models fail to accurately simulate droughts. Scientific American 2013 [cited]Available from: https://blogs.scientificamerican.com/the-curious-wavefunction/climate-change-models-fail-to-accurately-simulate-droughts/
- Kruger T, Geden O, Rayner S. Abandon hype in climate models. The Guardian. 2016. https://www.theguardian.com/science/political-science/2016/apr/26/abandon-hype-in-climate-models
You must be logged in to post a comment.