By Dilek Yildiz, Wittgenstein Center for Demography and Global Human Capital (IIASA, VID/ÖAW and WU), Vienna Institute of Demography, Austrian Academy of Sciences, International Institute for Applied Systems Analysis
Social media offers a promising source of data for social science research that could provide insights into attitudes, behavior, social linkages and interactions between individuals. As of the third quarter of 2017, Twitter alone had on average 330 million active users per month. The magnitude and the richness of this data attract social scientists working in many different fields with topics studied ranging from extracting quantitative measures such as migration and unemployment, to more qualitative work such as looking at the footprint of second demographic transition (i.e., the shift from high to low fertility) and gender revolution. Although, the use of social media data for scientific research has increased rapidly in recent years, several questions remain unanswered. In a recent publication with Jo Munson, Agnese Vitali and Ramine Tinati from the University of Southampton, and Jennifer Holland from Erasmus University, Rotterdam, we investigated to what extent findings obtained with social media data are generalizable to broader populations, and what constitutes best practice for estimating demographic information from Twitter data.
A key issue when using this data source is that a sample selected from a social media platform differs from a sample used in standard statistical analysis. Usually, a sample is randomly selected according to a survey design so that information gathered from this sample can be used to make inferences about a general population (e.g., people living in Austria). However, despite the huge number of users, the information gathered from Twitter and the estimates produced are subject to bias due to its non-random, non-representative nature. Consistent with previous research conducted in the United States, we found that Twitter users are more likely than the general population to be young and male, and that Twitter penetration is highest in urban areas. In addition, the demographic characteristics of users, such as age and gender, are not always readily available. Consequently, despite its potential, deriving the demographic characteristics of social media users and dealing with the non-random, non-representative populations from which they are drawn represent challenges for social scientists.
Although previous research has explored methods for conducting demographic research using non-representative internet data, few studies mention or account for the bias and measurement error inherent in social media data. To fill this gap, we investigated best practice for estimating demographic information from Twitter users, and then attempted to reduce selection bias by calibrating the non-representative sample of Twitter users with a more reliable source.
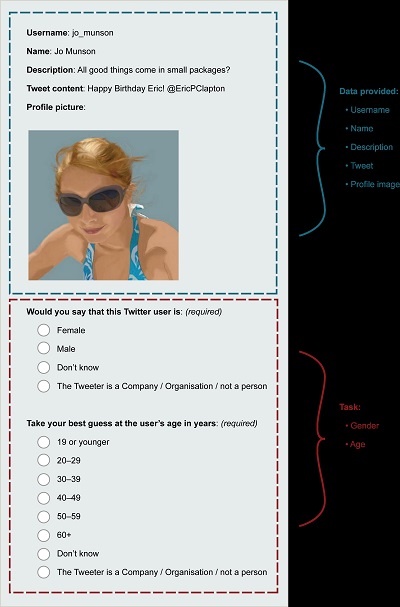
Exemplar of CrowdFlower task © Jo Munson.
We gathered information from 979,992 geo-located Tweets sent by 22,356 unique users in South-East England and estimated their demographic characteristics using the crowd-sourcing platform CrowdFlower and the image-recognition software Face++. Our results show that CrowdFlower estimates age more accurately than Face++, while both tools are highly reliable for estimating the sex of Twitter users.
To evaluate and reduce the selection bias, we ran a series of models and calibrated the non-representative sample of Twitter users with mid-year population estimates for South-East England from the UK Office of National Statistics. We then corrected the bias in age-, sex-, and location-specific population counts. This bias correction exercise shows promise for unbiased inference when using social media data and can be used to further reduce selection bias by including other sociodemographic variables of social media users such as ethnicity. By extending the modeling framework slightly to include an additional variable, which is only available through social media data, it is also possible to make unbiased inferences for broader populations by, for example, extracting the variable of interest from Tweets via text mining. Lastly, our methodology lends itself for use in the calculation of sample weights for Twitter users or Tweets. This means that a Twitter sample can be treated as an individual-level dataset for micro-level analysis (e.g., for measuring associations between variables obtained from Twitter data).
Reference:
Yildiz, D., Munson, J., Vitali, A., Tinati, R. and Holland, J.A. (2017). Using Twitter data for demographic research, Demographic Research, 37 (46): 1477-1514. doi: 10.4054/DemRes.2017.37.46
Note: This article gives the views of the author, and not the position of the Nexus blog, nor of the International Institute for Applied Systems Analysis.
You must be logged in to post a comment.